1. Introduction
Coronavirus Disease 2019 (COVID-19) is an infectious condition caused by a recently discovered coronavirus. In early March 2020, COVID-19 was declared a pandemic by the World Health Organization [
1]. Since its emergence and spread across the globe, the pandemic has been among the greatest disasters in decades, with more than one hundred million confirmed cases, several million deaths, and myriad serious socio-economic, political, and other impacts.
Although COVID-19 has been a global crisis, respecting no boundaries, the burden and cases in multiple African countries have been lower than was first feared or expected. Numerous countries across the continent experienced exponential growth in cases much later than other regions of the world; they have consistently confirmed fewer cases per capita [
2].
Africa, as a continent, has generally been successful since reporting its first case in February 2020; however, individual countries have experienced COVID-19 epidemics of vastly different scales and intensities. For instance, some countries, such as South Africa, Tunisia, and Egypt, have reported a far larger number of COVID-19 cases and deaths than others. The reason for considerable cross-country variability across the continent remains unclear. Accordingly, the present study examined the possible country-level factors associated with COVID-19 in Africa. Using multiple regression analysis on a unique cross-sectional dataset covering 54 African countries, essential characteristics linked with COVID-19-induced deaths were investigated.
Significantly, the present study enriched and contributed to the cumulative understanding of salient country-level factors associated with COVID-19-related outcomes. Furthermore, it helped to broaden awareness and provide useful insights concerning COVID-19 in Africa; a vast, complex continent of 54 diverse countries and over 1.3 billion individuals, i.e., received comparatively limited coverage and less research attention. Additionally, while many analyses have examined single or only limited factors that may potentially be associated with COVID-19, the present study explored a considerably larger number of characteristics; thus, offering a wide-ranging, more comprehensive analysis.
The rest of the paper unfolds as follows. The next section provides an overview of the methods, with an outline of the design, a description of the data examined, and details about sources. Subsequently, the results are presented, followed by the discussion section. The final section concludes, notes limitations, and recommends possible areas for future research.
2. Materials and Methods
This cross-sectional study covered 54 African countries. The required data were collected from various open-access databases. An array of demographic, political, socio-economic, and health-related indicators were selected for exploration and inclusion; they were largely guided by emerging empirical findings, the rapidly evolving literature, relevant theoretical frameworks, and data availability [
3,
4,
5,
6]. The study variables consisted of the following:
COVID-19 burden: the COVID-19 burden on individual African countries was measured concerning cumulative total deaths per million population. The necessary data were collected from the ‘Our World in Data’ open-access database; the cumulative total COVID-19 deaths were considered per million accurate as of 11 April 2021 [
7].
Gross Domestic Product (GDP) per capita: GDP is among the most popular and widely used proxy measures of economic productivity, output, and wellbeing. This variable presents a country’s GDP divided by its total population. The relevant data were presented in 2010 in constant US dollars and transformed (natural log) to attenuate for skew. The required data were collected from the World Bank’s World Development Indicators (WDI) database; it provides comprehensive cross-country comparable data on development [
8].
Global connectivity: frequently used as a proxy measure for global connectivity, mobility or the movement of individuals, and international integration, this variable represents the sum of a country’s imports and exports of goods and services divided by its GDP. The necessary data were gathered from the WDI database and transformed (natural log) to attenuate for skew [
9].
National health system: since conventional measures of national health system capacity (e.g., physicians per 1000 population, intensive care unit capacity, or hospital beds per 1000 population) are plagued by missing data, immunization coverage was used as a proxy. National Diphtheria, Tetanus, and Pertussis (DTP) immunization coverage is widely considered as a standard measure of the strength of national health systems; particularly since delivery entails 3 contacts with the health system at appropriate times. Additionally, it is part of routine national vaccination programs rather than campaigns. DTP immunization coverage is defined as the frequency of children aged 12-23 months, who have received vaccinations before 12 months or at any time before the survey. The required data were collected from the WDI database [
10,
11,
12].
Population density: collected from the WDI dataset, the population density was measured as the total population of a country divided by its total land area in square kilometers [
13]. Population age structure: this characteristic, gathered from the World Population Prospects database, presents the median age of the population of a country; it divides the total population into two equal parts. Accordingly, there are as many individuals aged above the median as there are below it. Figures are expressed in years [
14].
Polity: data for polity were collected from the extremely popular and widely used Polity5 Project database. This platform codes states’ political regime and authority characteristics. For each country, a “polity score” is determined, ranging from +10 (reflecting strongly democratic states) to -10 (reflecting strongly autocratic states). Scores are scaled based on an evaluation of democratic and autocratic characteristics and the elements of regimes, including elections, the nature of political participation, and the extent of checks on executive authority [
15].
Women’s leadership and representation: this variable presents the number of seats held by women members in the single or lower chambers of national parliaments, expressed as a percentage of all occupied seats. It is calculated by dividing the total number of seats occupied by women by the total number of seats in the national parliament. The necessary data were retrieved from the WDI dataset [
16].
The obtained data were extracted in Microsoft Excel format per characteristic. Then, they were imported into SPSS for statistical analysis. For the preliminary investigation of variables, descriptive statistics and correlations were examined. Subsequently, regression analysis was conducted, allowing a better understanding of relationships between variables and variations in COVID-19-induced deaths.
3. Results
Table 1 summarizes the collected descriptive statistics.
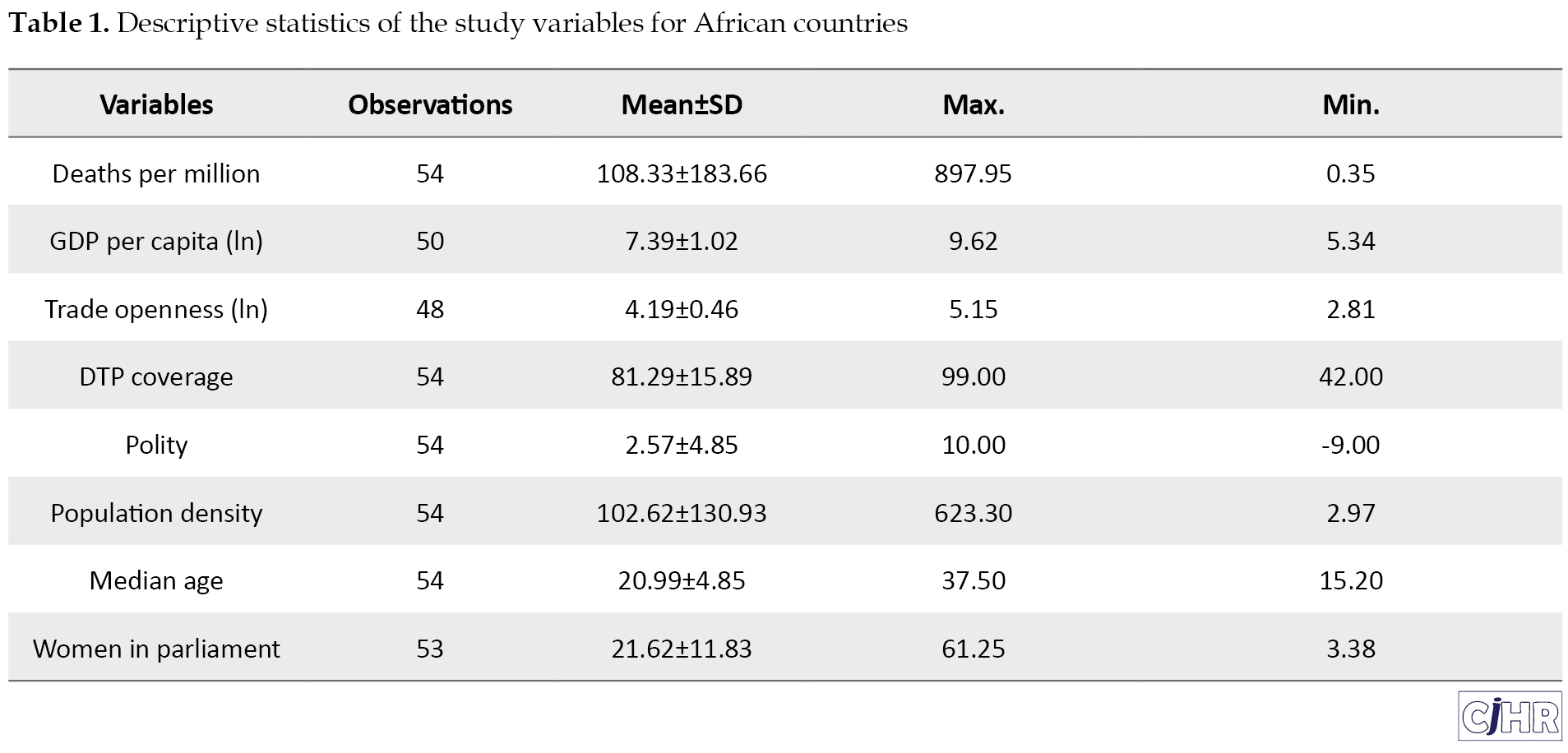
For the examined 54 African countries, the mean cumulative total COVID-19-related deaths per million equaled 108.33. For GDP per capita (natural log), the mean score was calculated as 7.39; however, for connectivity, the same was measured through trade openness (natural log), which equaled 4.19. The mean DTP coverage was approximately 81, while the mean polity score was approximately 2.57. Population density presented a mean value of about 102.62; however, the mean scores for median age and percentage of parliamentary seats held by women were measured as 20.99 and 21.62, respectively.
Table 2 presents a correlation matrix of the examined variables.
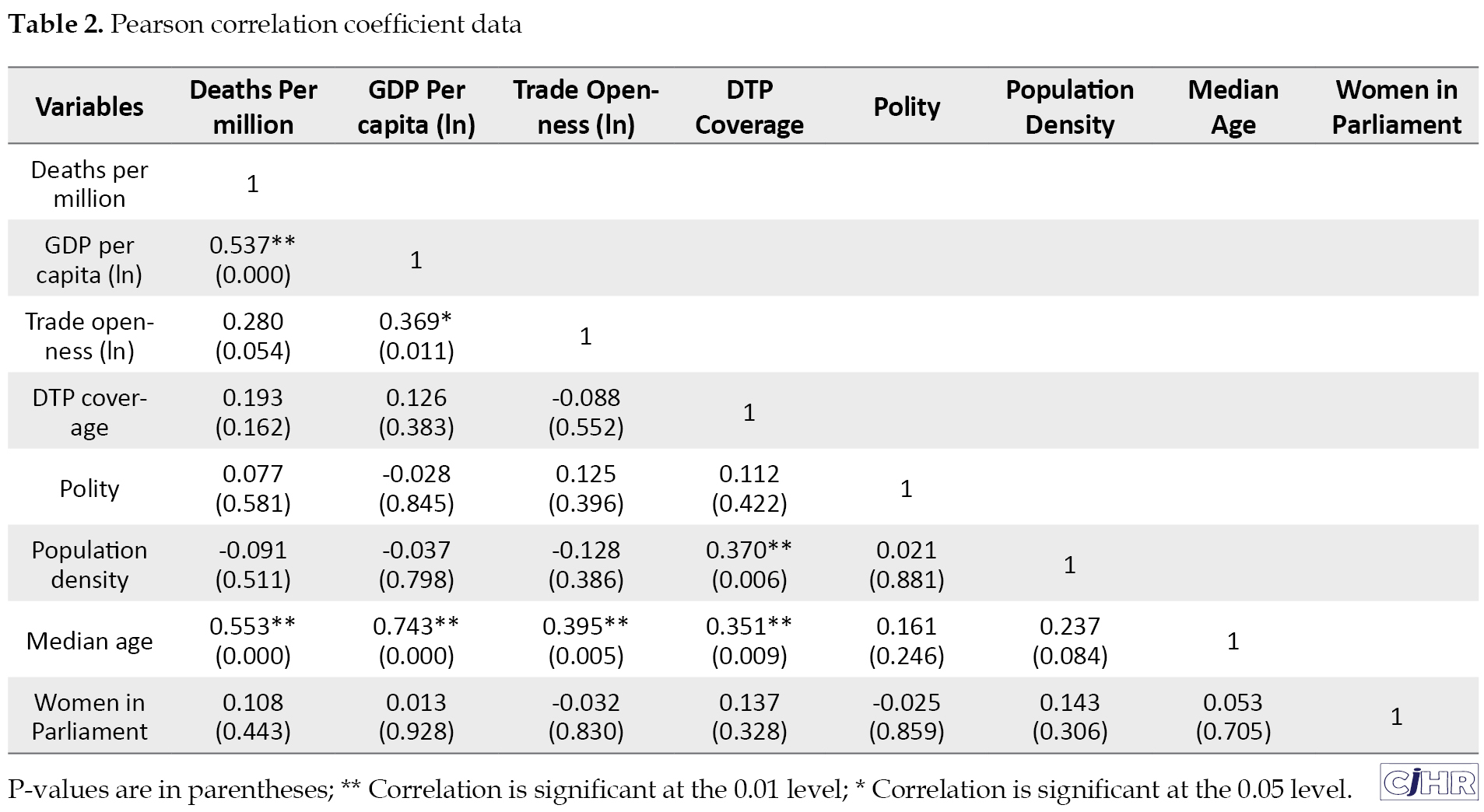
The relevant results illustrated a moderate, statistically significant positive correlation between deaths per million of the population and GDP per capita (r=0.537, P=0.000), as well as a moderate, statistically significant positive correlation between deaths per million population and median age (r=0.553, P=0.000). Thus, the achieved results indicated that a greater GDP per capita was positively associated with more deaths per million. Similarly, as the value of the median age of a country increased, so did the rate of deaths per million.
The regression analysis data are presented in
Table 3.
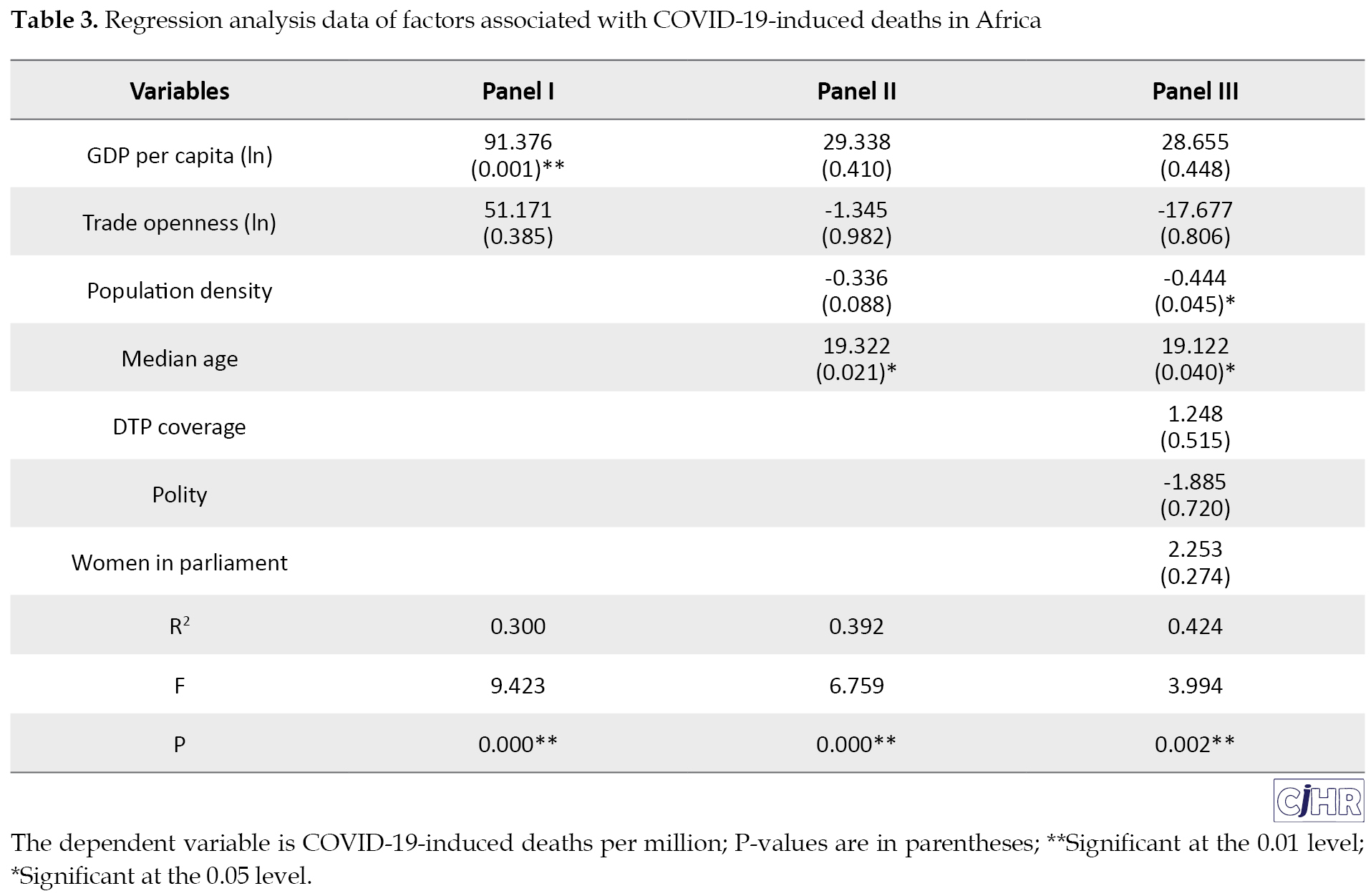
The explored variables were entered in several progressive stages, based upon socio-economic, political, demographic, and health-related dimensions to better examine their possible association with COVID-19-induced deaths per million. Panel I explored GDP per capita and connectivity. The regression analysis results indicated that panel I explained 30% of the variance (R2=0.300), i.e., a significant predictor of deaths per million, [F(2, 44)= 9.423, P=0.000]. While GDP per capita significantly contributed (B=91.376, P=0.001), connectivity did not (B=51.171, P=0.385) contribute to such rates.
Panel II retained GDP per capita and connectivity while adding population density and median age. The present study findings revealed that panel II explained 39.2% of the variation of deaths per million (R2=0.392), i.e., significantly useful in explaining deaths per million [F(4, 42)=6.759, P=0.000]. The estimates highlighted that median age was positively associated with deaths per million at the 5% level (B=19.322, P=0.021). In other words, as median age increases by one unit, COVID-19 deaths per million elevate by 19.322 units. However, the other variables included failure to demonstrate a statistically significant relationship with deaths per million.
Eventually, panel III, which retains the previous variables, also adds DTP coverage, polity, and the percentage of parliamentary seats held by women, explained approximately 42.4% of the variation in deaths per million (R2=0.424). The relevant results illustrated that panel III was significantly useful in explaining deaths per million [F(7, 38)=3.994, P=0.002]. Median age reflected a positive association with deaths per million at the 5% level (B = 19.122, P=0.040); population density also contributed significantly, being reversely associated with deaths per million at the 5% level (B=-0.444, P=0.045). Thus, as median age increases, so does COVID-19-related deaths per million. In contrast, as population density increases, deaths per million decrease. However, the various other included variables made no significant contribution in this respect.
4. Discussion
The present research highlighted several essential findings and provided useful insights. For instance, a large body of work revealed that overcrowding and greater population density can facilitate or ease the transmission of an array of infectious diseases and enhance their incidence [
17,
18,
19,
20,
21]. Given that COVID-19 is mainly spread through close contact with infected individuals [
22,
23,
24], empirical work has explored how population density may be associated with the national COVID-19 burden. Wong and Li (2020) argued that population density is an effective predictor of cumulative infection cases in the United States at the county level [
25]; however, research in Bangladesh has found a strong positive correlation between population density and COVID-19 [
26]. Additionally, other work has identified that densely populated countries presented higher rates of coronavirus infection cases [
27,
28].
Contrary to such data, the present study outlined that population density was negatively associated with COVID-19-induced deaths per million. Perhaps counterintuitive, this finding generally aligns with previous research, indicating that density may be associated with fewer COVID-19 cases or relevant deaths. In other words, higher density areas enjoy a better healthcare infrastructure, higher-grade facilities, further or better staff, and specialized services, faster response times, as well as a greater concentration of intensive-care beds and other resources; all of which can help reduce COVID-19 cases, severity, and related deaths [
29,
30].
Notably, the present study findings demonstrated that median age is positively associated with COVID-19-related deaths; these data were in line with those of other studies suggesting that age is related to COVID-19 burden [
31,
32,
33,
34]. Older population groups have had strikingly higher COVID-19 mortality rates compared to younger groups, globally. Besides, younger age is associated with a lower odds of severe COVID-19 cases [
35,
36,
37]. COVID-19 can infect and kill the young; however, they generally experience much milder symptoms, compared to older populations (who undergo age-related physiological changes & have reduced immunity or comorbidities). Moreover, there are very few severe cases and deaths from COVID-19 among children and young adolescents [
38,
39,
40,
41].
Some research has indicated that global connectivity and international air traffic were significant facilitators in the early global transmission of COVID-19. Additionally, they played a role in the transmission of cases from highly infected countries. Lau et al. (2020), for instance, suggested that the total number of flight routes and passenger volume were relevant risk factors for COVID-19 spread [
42]. Furthermore, in an investigation of 34 countries reporting international flights to 154 different countries, Keita (2020) reported that less-connected countries registered first infection cases significantly later than more-connected ones; thus, travel restrictions could help to slow the spread of infection [
43]. In contrast, the present study revealed that global connectivity was not associated with COVID-19-induced deaths.
The present study findings regarding GDP per capita and the national health system, with DTP coverage as a proxy, were interesting. GDP and economic wealth have consistently been signified to be major determinants of health and development outcomes [
44,
45,
46,
47]. Additionally, evidence suggested that a country’s economic performance improves life expectancy, and declines adverse health outcomes and mortality rates [
48]. Emerging work on COVID-19 has found that economic factors may be associated with severity [
49], as well as the odds of fatality [
50]; however, national GDP per capita has been linked with a lower rate of new COVID-19 cases [
51,
52]. Similarly, there exists a large body of literature demonstrating that effective public healthcare systems are crucial in controlling disease, promoting development, and ensuring population health [
53,
54]. Furthermore, the COVID-19 pandemic has helped demonstrate the significance of robust, effective, and resilient public health sectors [
55,
56,
57].
GDP per capita and DTP coverage were not significantly impactful in the present study. This finding was in line with previous investigations signifying that countries’ wealth may not be the main or sole factor in health security and COVID-19 outcomes. Throughout the COVID-19 pandemic, multiple high GDP countries, with well-established healthcare systems have been among those most severely hit; however, a considerable number of lower-income countries have registered lower COVID-19 incidence and mortality rates. Notwithstanding numerous challenges, lower-income countries may be able to efficiently allocate their scarce, limited resources, and effectively implement or enforce various policies and guidelines [
58,
59,
60].
The possible impact of political regime type or the nature of a country’s political system on COVID-19 response and outcomes remains a prominent topic of debate and analysis since the outbreak and global spread of COVID-19. Generally, two broad and opposing views can be distinguished. Within autocracies, while centralized power may allow quick, decisive action, the tendency to maintain secrecy means information can be suppressed and critical problems ignored. Additionally, the hostility of autocracies toward independent or contrarian voices and civil society may hinder effective public health responses; civil society may support the collection and distribution of accurate, timely information, or delivery of resources and care. The greater transparency of democracies promotes a freer flow of information and complicates covering up threats or crises; the decentralization of power, i.e., intrinsic to democracies may contribute to a slow and potentially ineffective response. Moreover, democratic countries are characterized by laissez-faire administrative systems; citizens are potentially less obliged to strictly follow and implement public health guidelines or measures that may impinge on the freedom of movement and other rights [
61,
62,
63].
The present study revealed that political regime is not associated with COVID-19 deaths. This result aligns with the fact that no strong or clear consensus exists within the evolving literature. COVID-19 has not differentiated between democracies and autocracies on the continent; a large, sole focus on the type or nature of the government system may obscure understanding of other relevant factors. Rather than the particular type of political regime, several specific aspects or characteristics of countries, such as population density and age, seem to be more important for COVID-19-related outcomes.
Another persistent question throughout the COVID-19 pandemic was whether women leaders around the globe have managed the pandemic differently or more competently than their male counterparts [
64,
65]. For instance, Johnson and Williams (2020) argued that while male leaders have historically drawn on their traditionally-defined role as the head of household to demonstrate masculine protectionism toward citizens, women leaders may draw upon their traditionally-defined motherly role. For instance, the individual in the family who conventionally cares for the sick member presents feminine protectionism [
66].
The literature on attitudes to risk and uncertainty offers possible explanations for gender-wise differences. For instance, there are indications that women, including those within leadership positions, are more risk- and loss-averse than men [
67,
68,
69]. Thus, they may be less willing to accept health risks and more prone to respond quickly or lockdown earlier. Additionally, a greater proportion of women in national parliament might be linked with the adoption of different solutions; for instance, a higher frequency of women in the national legislature has been associated with increased spending on public health [
70].
Of note, greater diversity and gender balance in leadership have been outlined as an essential requirement in successful pandemic preparedness and responses [
71]. Moreover, a study on COVID-19 deaths in the USA found that states with male governors encountered further COVID-19 deaths, compared to the states with women governors [
72]. Similarly, after examining various public health metrics to measure the control of the COVID-19 epidemic in women- versus men-led countries globally, Purkayastha, Salvatore, and Mukherjee (2020) concluded that although not statistically significant, countries that are led by females perform better respecting public health metrics for controlling the spread of the COVID-19 pandemic, compared to the countries led by males [
73].
However, the present study findings on the percentage of seats in parliament held by women were not statistically significant and consistent with the growing body of literature, indicating no statistical support for the hypothesis that men leaders have less competently and effectively responded to the COVID-19 pandemic than women leaders [
74,
75]. Similar to political regime type, it is likely not so much the particular gender of those in positions of leadership, but the attributes and characteristics of the countries that they administer, i.e., most consequential for COVID-19-related outcomes.
5. Conclusion
Since its emergence and spread across the globe, the COVID-19 pandemic has been one of the world’s most devastating crises in numerous decades. Africa’s COVID-19-related figures have been far lower than originally expected; however, there has been striking country-to-country variation in the burden across the continent. The present study examined the factors related to COVID-19-induced deaths at the country level. The obtained data illustrated that COVID-19-related deaths per million were positively associated with median age and reversely correlated with population density. Other explored factors, guided by emerging empirical findings, the rapidly evolving literature, and relevant theoretical frameworks, such as GDP per capita, connectivity, DTP coverage, percentage of seats in parliament held by women, and political regime type, demonstrated no statistical significance in this respect.
While the present study helps broaden awareness, improve understanding, and provide useful insights regarding COVID-19 on the continent, several caveats remain to keep in mind. First, with African countries lagging far behind much of the world concerning vaccination rollout and as the pandemic continues to unfold and evolve on the continent, COVID-19 figures and trends are prone to change; leaving the possibility for shifts in findings and conclusions presented here. Second, while the current study has explored several country-level factors potentially related to cross-country variability in COVID-19 outcomes, there remain other characteristics that may be investigated to better understand variations, across and within countries, such as humidity or climate. Finally, the present study was cross-sectional; thus, findings may be different in other time frames. Moreover, future studies may examine time-series data to provide longitudinal evaluations.
Ethical Considerations
Compliance with ethical guidelines
There were no ethical considerations to be considered in this research.
Funding
This research did not receive any grant from funding agencies in the public, commercial, or non-profit sectors.
Conflict of interest
The authors declared no conflicts of interest.
References
- World Health Organization. WHO director-general's opening remarks at the media briefing on COVID-19 [Internet]. 2020 [Updated 2020 March 11]. Available from: https://www.who.int/director-general/speeches/detail/who-director-general-s-opening-remarks-at-the-media-briefing-on-covid-19---11-march-2020
- Partnership for Evidence-Based Response to COVID-19 (PERC). Responding to COVID-19 in Africa: Using data to find a balance - Part II [Internet]. 2020 [Updated 2021 July]. Available from: https://www.afro.who.int/publications/responding-covid-19-africa-using-data-find-balance-part-ii
- Baum F, Freeman T, Musolino C, Abramovitz M, De Ceukelaire W, Flavel J, et al. Explaining Covid-19 performance: What factors might predict national responses? BMJ. 2021; 372:n91. [DOI:10.1136/bmj.n91] [PMID] [PMCID]
- Gesesew HA, Koye DN, Fetene DM, Woldegiorgis M, Kinfu Y, Geleto AB, et al. Risk factors for COVID-19 infection, disease severity and related deaths in Africa: A systematic review. BMJ Open. 2021; 11(2):e044618. [DOI:10.1136/bmjopen-2020-044618] [PMID] [PMCID]
- Liang LL, Tseng CH, Ho HJ, Wu CY. Covid-19 mortality is negatively associated with test number and government effectiveness. Sci Rep. 2020; 10(1):12567. [DOI:10.1038/s41598-020-68862-x] [PMID] [PMCID]
- Musa HH, Musa TH, Musa IH, Musa IH, Ranciaro A, Campbell MC. Addressing Africa's pandemic puzzle: Perspectives on COVID-19 transmission and mortality in sub-Saharan Africa. Int J Infect Dis. 2021; 102:483-8. [DOI:10.1016/j.ijid.2020.09.1456] [PMID] [PMCID]
- Ritchie H, Ortiz-Ospina E, Beltekian D, Mathieu E, Hasell J, Macdonald B, et al. Coronavirus pandemic (COVID-19) [Internet]. 2020 [Updated 2021 July 24]. Available from: https://ourworldindata.org/coronavirus
- The World Bank. GDP per capita (constant 2010 US$) [Internet]. 2020 [Updated 2020]. Available from: https://data.worldbank.org/indicator/NY.GDP.PCAP.KD
- The World Bank. Trade (% of GDP) [Internet]. 2020 [Updated 2020]. Available from: https://data.worldbank.org/indicator/NE.TRD.GNFS.ZS
- Bos E, Batson A. Using immunization coverage rates for monitoring health sector performance: measurement and interpretation issues. Washington, DC.: World Bank; 2000. https://openknowledge.worldbank.org/handle/10986/13800
- Ikilezi G., Augusto OJ, Sbarra A, Sherr K, Dieleman JL, Lima SS. Determinants of geographical inequalities for DTP3 vaccine coverage in sub-Saharan Africa. Vaccine. 2020; 38(18):3447-54. [DOI:10.1016/j.vaccine.2020.03.005] [PMID]
- The World Bank. Immunization, DPT (% of children ages 12-23 months) [Internet]. 2019 [Updated 2019]. Available from: https://data.worldbank.org/indicator/SH.IMM.IDPT
- The World Bank. Population density (people per sq. km of land area) [Internet]. 2018 [Updated 2018]. Available from: https://data.worldbank.org/indicator/EN.POP.DNST
- United Nations, Department of Economic and Social Affairs, Population Division. World population prospects 2019 [Internet]. 2019 [Updated 2019]. Available from: https://population.un.org/wpp/Download/Files/1_Indicators%20(Standard)/EXCEL_FILES/1_Population/WPP2019_POP_F05_MEDIAN_AGE.xlsx
- Marshall MG, Gurr TR. Polity5: Political regime characteristics and transitions, 1800-2018 [Internet]. 2020 [Updated 2020 April 23]. Available from: http://www.systemicpeace.org/inscr/p5manualv2018.pdf
- The World Bank. Proportion of seats held by women in national parliaments (%) [Internet]. 2020 [Updated 2020]. Available from: https://data.worldbank.org/indicator/SG.GEN.PARL.ZS
- Jones KE, Patel NG, Levy MA, Storeygard A, Balck D, Gittleman JL, et al. Global trends in emerging infectious diseases. Nature. 2008; 451(7181):990-3. [DOI:10.1038/nature06536] [PMID] [PMCID]
- Osei FB, Duker AA. Spatial and demographic patterns of cholera in Ashanti region - Ghana. Int J Health Geogr. 2008; 7:44. [DOI:10.1186/1476-072X-7-44] [PMID] [PMCID]
- Tarwater PM, Martin CF. Effects of population density on the spread of disease. Complexity. 2001; 6(6):29-36. [DOI:10.1002/cplx.10003]
- Velimirovic B, Subramanian M, Sadek F. Socio-economic and environmental factors and human health example of cholera El Tor in Manila. Zentralbl Bakteriol Orig B. 1975; 160(1):1-27. [PMID]
- World Health Organization. Scabies [Internet]. 2020 [Updated 2020 August 16]. Available from: https://www.who.int/news-room/fact-sheets/detail/scabies
- Chu DK, Akl EA, Duda S, Solo K, Yaacoub S, Schunemann HJ, et al. Physical distancing, face masks, and eye protection to prevent person-to-person transmission of SARS-CoV-2 and COVID-19: a systematic review and meta-analysis. Lancet. 2020; 395(10242):1973-87. [DOI:10.1016/S0140-6736(20)31142-9]
- Marín-García D, Moyano-Campos JJ, Bienvenido-Huertas JD. Distances of transmission risk of COVID-19 inside dwellings and evaluation of the effectiveness of reciprocal proximity warning sounds. Indoor Air. 2021; 31(2):335-47. [DOI:10.1111/ina.12738] [PMID]
- World Health Organization. Coronavirus disease (COVID-19): How is it transmitted? [Internet]. 2020 [Updated 2021 April 30]. Available from: https://www.who.int/news-room/q-a-detail/coronavirus-disease-covid-19-how-is-it-transmitted
- Wong DWS, Li Y. Spreading of COVID-19: Density matters. PLoS One. 2020; 15(12):e0242398. [DOI:10.1371/journal.pone.0242398] [PMID] [PMCID]
- Alam MZ. Is population density a risk factor for communicable diseases like COVID-19? A case of Bangladesh. Asia Pac J Public Health. 2021. [DOI:10.1177/1010539521998858] [PMID] [PMCID]
- Liu J, Liao X, Qian Sh, Yuan J, Wang F, Liu Y, et al. Community transmission of severe acute respiratory syndrome Coronavirus 2, Shenzhen, China, 2020. Emerg Infect Dis. 2020; 26(6):1320-3. [DOI:10.3201/eid2606.200239] [PMID] [PMCID]
- Rocklöv J, Sjödin H. High population densities catalyse the spread of COVID-19. J Travel Med. 2020; 27(3):taaa038. [DOI:10.1093/jtm/taaa038] [PMID] [PMCID]
- Hamidi Sh, Sabouri S, Ewing R. Does density aggravate the COVID-19 pandemic? J Am Plann Assoc. 2020; 86(4):495-509. [DOI:10.1080/01944363.2020.1777891]
- Ji Y, Ma Zh, Peppelenbosch MP, Pan Q. Potential association between COVID-19 mortality and health-care resource availability. Lancet Glob Health. 2020; 8(4):E480. [DOI:10.1016/S2214-109X(20)30068-1]
- Lulbadda KT, Kobbekaduwa D, Guruge ML. The impact of temperature, population size and median age on COVID-19 (SARS-CoV-2) outbreak. Clin Epidemiol Glob Health. 2021; 9:231-6. [DOI:10.1016/j.cegh.2020.09.004] [PMID] [PMCID]
- Toker K, Gözübol Zİ. The relationship between median age and death cases during the Coronavirus Disease 2019 (COVID-19) pandemic: The mediating role of current health expenditure. Bezmialem Sci. 2020; 8(Suppl 2):1-6. [DOI:10.14235/bas.galenos.2020.4846]
- Velasco JM, Tseng WC, Chang CL. Factors affecting the cases and deaths of COVID-19 victims. Int J Environ Res Public Health. 2021; 18(2):674. [DOI:10.3390/ijerph18020674] [PMID] [PMCID]
- Wang D, Hu B, Hu Ch, Zhu F, Liu X, Zhang J, et al. Clinical characteristics of 138 hospitalised patients with 2019 novel coronavirus-infected pneumonia in Wuhan, China. JAMA. 2020; 323(11):1061-9. [DOI:10.1001/jama.2020.1585] [PMID] [PMCID]
- Brooke J, Jackson D. Older people and COVID-19: Isolation, risk and ageism. J Clin Nurs. 2020; 29(13-14):2044-6. [DOI:10.1111/jocn.15274] [PMID]
- Li X, Xu Sh, Yu M, Wang K, Tao Y, Zhou Y, et al. Risk factors for severity and mortality in adult COVID-19 inpatients in Wuhan. J Allergy Clin Immunol. 2020; 146(1):110-8. [DOI:10.1016/j.jaci.2020.04.006] [PMID] [PMCID]
- Yanez ND, Weiss NS, Romand JA, Treggiari MM. COVID-19 mortality risk for older men and women. BMC Public Health. 2020; 20(1):1742. [DOI:10.1186/s12889-020-09826-8] [PMID] [PMCID]
- Shi Y, Yu X, Zhao H, Wang H, Zhao R, Sheng J. Host susceptibility to severe COVID-19 and establishment of a host risk score: findings of 487 cases outside Wuhan. Crit Care. 2020; 24(1):108. [DOI:10.1186/s13054-020-2833-7] [PMID] [PMCID]
- Snape MD, Viner RM. COVID-19 in children and young people. Science. 2020; 370(6514):286-8. [DOI:10.1126/science.abd6165] [PMID]
- Starke KR, Petereit-Haack G, Schubert M, Kämpf D, Schliebner A, Hegewald J, et al. The age-related risk of severe outcomes due to COVID-19 infection: A rapid review, meta-analysis, and meta-regression. Int J Environ Res Public Health. 2020; 17(16):5974. [DOI:10.3390/ijerph17165974] [PMID] [PMCID]
- Wang XQ, Song G, Yang Zh, Chen RJ, Zheng YL, Hu HY, et al. Association between ageing population, median age, life expectancy and mortality in Coronavirus Disease (COVID-19). Aging. 2020; 12(24):24570-8. [DOI:10.18632/aging.104193] [PMID] [PMCID]
- Lau H, Khosrawipour V, Kocbach P, Mikolajczyk A, Ichii H, Zacharski M, et al. The association between international and domestic air traffic and the Coronavirus (COVID-19) outbreak. J Microbiol Immunol Infect. 2020; 53(3):467-72. [DOI:10.1016/j.jmii.2020.03.026] [PMID] [PMCID]
- Keita S. Air passenger mobility, travel restrictions, and the transmission of the COVID-19 pandemic between countries. Covid Economics. 2020; (9):77-96. https://www.iab.de/897/section.aspx/Publikation/k200429301
- Brenner MH. Commentary: Economic growth is the basis of mortality rate decline in the 20th century--experience of the United States 1901-2000. Int J Epidemiol. 2005; 34(6):1214-21. [DOI:10.1093/ije/dyi146] [PMID]
- Filmer D, Pritchett L. The impact of public spending on health: Does money matter? Soc Sci Med. 1999; 49(10):1309-23. [DOI:10.1016/S0277-9536(99)00150-1]
- Pritchett L, Summers LH. Wealthier is healthier. J Hum Resour. 1996; 31(4):841-68. [DOI:10.2307/146149]
- Swift R. The relationship between health and GDP in OECD countries in the very long run. Health Econ. 2011; 20(3):306-22. [DOI:10.1002/hec.1590] [PMID]
- Berkman LF, Kawachi I, Glymour MM, editors. Social epidemiology. Oxford: Oxford University Press; 2014. [DOI:10.1093/med/9780195377903.001.0001]
- Williamson EJ, Walker AJ, Bhaskaran K, Bacon S, Bates Ch, Morton CE, et al. Factors associated with COVID-19-related death using Open SAFELY. Nature. 2020; 584(7821):430-6. [DOI:10.1038/s41586-020-2521-4] [PMID] [PMCID]
- Banik A, Nag T, Chowdhury SR, Chatterjee R. Why do COVID-19 fatality rates differ across countries? An explorative cross-country study based on select indicators. Glob Bus Rev. 2020; 21(3):607-25. [DOI:10.1177/0972150920929897]
- Lippi G, Henry BM, Mattiuzzi C, Bovo Ch. The death rate for COVID-19 is positively associated with gross domestic products. Acta Biomed. 2020; 91(2):224-5. [DOI:10.23750/abm.v91i2.9514] [PMID] [PMCID]
- Pardhan Sh, Drydakis N. Associating the change in new COVID-19 cases to GDP per capita in 38 European countries in the first wave of the pandemic. Front Public Health. 2021; 8:582140. [DOI:10.3389/fpubh.2020.582140] [PMID] [PMCID]
- Macinko J, Starfield B, Erinosho T. The impact of primary healthcare on population health in low- and middle-income countries. J Ambul Care Manage. 2009; 32(2):150-71. [DOI:10.1097/JAC.0b013e3181994221] [PMID]
- Starfield B. Is strong primary care good for health outcomes? In: Griffin J, editor. The Future of Primary Care. London: Office of Health Economics; 1996. pp. 18-29. https://www.ohe.org/publications/future-primary-care
- Dunlop C, Howe A, Li D, Allen LN. The coronavirus outbreak: The central role of primary care in emergency preparedness and response. BJGP Open. 2020; 4(1):bjgpopen20X101041. [DOI:10.3399/bjgpopen20X101041] [PMID] [PMCID]
- Lal A, Erondu NA, Heymann DL, Gitahi G, Yates R. Fragmented health systems in COVID-19: Rectifying the misalignment between global health security and universal health coverage. Lancet. 2021; 397(10268):61-7. [DOI:10.1016/S0140-6736(20)32228-5]
- Lauriola P, Martín-Olmedo P, Leonardi GS, Bouland C, Verheij R, Dückers MLA, et al. On the importance of primary and community healthcare in relation to global health and environmental threats: Lessons from the COVID-19 crisis. BMJ Glob Health. 2021; 6(3):e004111. [DOI:10.1136/bmjgh-2020-004111] [PMID] [PMCID]
- Chowdhury AZ, Jomo KS. Responding to the COVID19 pandemic in developing countries: Lessons from selected countries of the global south. Development. 2020; 63(2):162-71. [DOI:10.1057/s41301-020-00256-y] [PMID] [PMCID]
- Condo J, Uwizihiwe JP, Nsanzimana S. Learn from Rwanda's success in tackling COVID-19. Nature. 2020; 581(7809):384. [DOI:10.1038/d41586-020-01563-7] [PMID]
- Trevisan M, Le LC, Le AV. The COVID-19 pandemic: A view from Vietnam. Am J Public Health. 2020; 110(8):1152-3. [DOI:10.2105/AJPH.2020.305751] [PMID] [PMCID]
- Alon I, Farrell M, Li Sh. Regime type and COVID-19 response. FIIB Bus Rev. 2020; 9(3):152-60. [DOI:10.1177/2319714520928884]
- Carothers T, Wong D. Authoritarian weaknesses and the pandemic [Internet]. 2020 [Updated 2020 August 11]. Available from: https://carnegieendowment.org/2020/08/11/authoritarian-weaknesses-and-pandemic-pub-82452
- Stasavage D. Democracy, autocracy, and emergency threats: Lessons for COVID-19 from the last thousand years. Int Organ. 2020; 74(S1):E1-17. [DOI:10.1017/S0020818320000338]
- Anderson C. Why do women make such good leaders during COVID-19? [Internet]. 2020 [Updated 2020 April 19]. Available from: https://www.forbes.com/sites/camianderson1/2020/04/19/why-do-women-make-such-good-leaders-during-covid-19/?sh=363cbf9742fc
- Piazza KS, Diaz G. Light in the midst of chaos: COVID-19 and female political representation. World Dev. 2020; 136:105125. [DOI:10.1016/j.worlddev.2020.105125] [PMID] [PMCID]
- Johnson C, Williams B. Gender and political leadership in a time of COVID. Polit Gend. 2020; 16(4):943-50. [DOI:10.1017/S1743923X2000029X] [PMCID]
- Byrnes JP, Miller DC, Schafer WD. Gender differences in risk taking: A meta-analysis. Psychol Bull. 1999; 125(3):367-83. [DOI:10.1037/0033-2909.125.3.367]
- Charness G, Gneezy U. Strong evidence for gender differences in risk taking. J Econ Behav Organ. 2012; 83(1):50-8. [DOI:10.1016/j.jebo.2011.06.007]
- Croson R, Gneezy U. Gender differences in preferences. J Econ Lit. 2009; 47(2):448-74. [DOI:10.1257/jel.47.2.448]
- Clayton A, Zetterberg P. Quota shocks: Electoral gender quotas and government spending priorities worldwide. J Polit. 2018; 80(3):916-32. [DOI:10.1086/697251]
- Meagher K, Singh NS, Patel P. The role of gender inclusive leadership during the COVID-19 pandemic to support vulnerable populations in conflict settings. BMJ Glob Health. 2020; 5(9):e003760. [DOI:10.1136/bmjgh-2020-003760] [PMID] [PMCID]
- Sergent K, Stajkovic AD. Women's leadership is associated with fewer deaths during the COVID-19 crisis: Quantitative and qualitative analyses of United States governors. J Appl Psychol. 2020; 105(8):771-83. [DOI:10.1037/apl0000577] [PMID]
- Purkayastha S, Salvatore M, Mukherjee B. Are women leaders significantly better at controlling the contagion during the COVID-19 pandemic? J Health Soc Sci. 2020; 5(2):231-40. [PMID] [PMCID]
- Aldrich AS, Lotito NJ. Pandemic performance: Women leaders in the COVID-19 crisis. Polit Gend. 2020; 16(4):960-7. [DOI:10.1017/S1743923X20000549] [PMCID]
- Windsor LC, Reinhardt GY, Windsor AJ, Ostergard R, Allen S, Burns C, et al. Gender in the time of COVID-19: Evaluating national leadership and COVID-19 fatalities. PLoS One. 2020; 15(12):e0244531. [DOI:10.1371/journal.pone.0244531] [PMID] [PMCID]